Tools and Techniques to Simplify Complex Data Analysis
Nowadays, there’s data everywhere. From online accounts to smartphones to sensors on gadgets – information gets collected on just about everything we do. Companies and scientists have access to giant piles of facts and figures. It’s awesome in some ways. But it also gets confusing quick.
How can anyone make sense of all those massive spreadsheets and databases? So much information can feel overwhelming. Luckily, there are tried and true techniques to tackle the complexity. Analysts can use different tools to simplify things step-by-step. The key is breaking big problems to simplifying complex data.
Why simplifying gets complicated
As data piles multiply from various business units and processes into intertwined structures, we strain to trace the metrics that matter most. Important patterns and performance drivers fade into complex model overgrowths confusing even to data scientists.
Without sound simplification, departments waste resources arguing over mismatched dashboard figures that should synchronously inform priorities. Technological capabilities to crystallize coherence from chaos help companies align.
Tools for simplifying complex data
1. Automated data mapping
Smart data mapping tools do the grunt work of untangling tangled web of massive databases so we humans can focus on big picture thinking. Just like intelligent assistants handle chores to free up our time, automated algorithms organize heaping data piles into neat flowcharts mapping the connections using nifty similarity measures and machine learning tricks. They handle heavy lifting so we can spend our energy making strategic sense of things.
2. Data modeling software
Dedicated modeling tools like SAS Data Manager and Talend integrate disparate sources into consolidated data lakes, filtering noise while retaining signals most indicative of performance outcomes, customer behavior, operational bottlenecks, and more. Guided modeling walks business leaders through constructing unified frameworks. This is one of the major tools used in analyzing and simplifying complex data.
3. Visual entity-relationship diagrams
ERD visualizations map out entities like customers, inventory, vehicles, etc., and relationships between them, such as service calls tied to assets. Intuitive network graphs replace complex spreadsheets, so we trace behavioral patterns and business process chains through flowing funnel depictions calibrated to key strategic KPIs.
4. Spreadsheet pivot tables
Even basic spreadsheet tools enable restructuring complex data reports into filtered tables, isolating metrics of interest across desired timeframes and segments. Play with pivot interactions until trends and outliers emerge from the fog.
5. Programming packages
Leading statistical programming platforms like Python, R, and Julia offer extensive libraries to ingest diverse data sets. As code handles source merging, validation, and restructuring behind the scenes, teams focus creativity on equation-driven modeling kits for custom measurement solutions.
Advanced analysis techniques for simplifying complex data
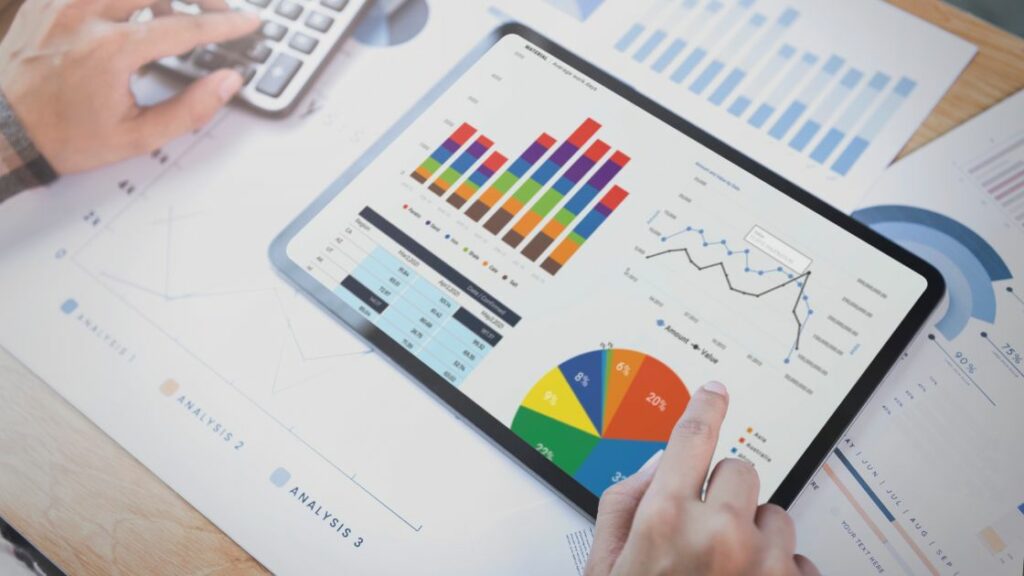
Visualizing complex data effectively transforms intricate datasets into comprehensible insights, aiding decision-making processes. Here are the techniques for simplifying complex data:
1. Machine learning prediction
Exploring rich data spaces like sentiment derived from customer support transcripts, predictive algorithms discover undisclosed correlations and classifications, translating qualitative feedback into quantitative strategic scores on brand affinity, churn risk, and more. By ingesting unstructured data like call center notes, emails, and social media, advanced ML models can unlock hidden insights on improving products, pricing, promotions, and overall customer lifetime value, even from conversational language. Moreover, machine learning prediction could be great for visualizing complex data.
2. Time series modeling
Understanding cyclical and seasonal effects when interpreting data avoids misleading short-term skews. Visual time series analysis frames demand changes, price fluctuations, and other trends across properly extended horizons via statistical techniques that eliminate noise, such as stock market volatility modeling. By incorporating historical context and future projections, time series models account for periodic micro-cycles and macro-cycles and one-off events to support more reliable forecasting and planning.
3. Text and image analysis
Converting unstructured data like social media video, scanned documents or computational photography into categorized text strings or pixel analysis prepares qualitative inputs for mathematical rigor so that vibrant but inscrutable customer voices get translated into measurable improvements. Layering machine learning on optical character recognition, speech-to-text transcription, semantic analysis, and computer vision models enables unlocking insights from qualitative data at unprecedented scale and specificity.
Strategies for democratizing your data
Now that data-wrangling workflows have transformed fragmented, complex data into simplified analytical models, business leaders should facilitate broader access to these insights across the organization.
a. Know your data
Catalog data dictionary metadata so that teams can easily reference definitions, sources, and calculation methodologies behind published figures as needed without chasing down creators.
b. Identify data silos
Map data ecosystem connections to link siloed analytics from sales, product, finance, and alternative perspectives into an enterprise data hub everyone accesses intuitively.
c. Select cloud data platforms
Curate self-serve cloud data access so business units apply governance while securely querying master analytics tailored to their decisions and self-educating through integrated discovery tools.
d. Establish tagging protocols
Classify data points by dimensions like product, region, and channel via embedded tags and metadata so consolidated views filter on-demand without complex joins as use cases evolve.
e. Track data lineage
Automate pipeline mapping from raw inputs onwards so that model stakeholders verify reliability. Catalogue transformations like smoothing, imputing missing values, etc., behind published charts to instill trust.
Conclusion
Companies must ensure data transparency and accessibility while balancing security and compliance. Democratization unlocks creativity as curiosity meets capability. With the right tools and governance orchestrating complexity, business insights flow freely, informing strategies with reliable clarity even at astronomical data scale.