Predictive analysis has become integral to how businesses operate and make strategic decisions. By analyzing current and historical data, predictive analysis aims to make statistically valid predictions about future outcomes and trends. This data-driven approach to forecasting provides organizations with actionable insights that can guide planning and optimize operations.
As data grows exponentially, predictive analytics is more vital than ever to stay ahead of the curve. This article will overview predictive analysis and outline key techniques leveraged today for data-based forecasting across various industries.
Core Concepts in Predictive Analysis
Data Collection
The first step in any predictive analysis is gathering relevant data. This may include sales numbers, customer information, website traffic, sensor readings, or other metrics that relate to what you want to predict. The more high-quality, recent data you have, the better your analysis can reflect current conditions when making data predictions.
You don’t necessarily need massive datasets, either. Even analyzing a few months of sales data could spot trends that translate into better next month’s production forecasting. The key is collecting accurate, complete data that connects to the target variable you want to predict. Tracking data over time rather than at just a single point is also crucial for uncovering patterns.
Data Cleaning and Preparation
Before statistical modeling, some data prep is required. This includes steps like handling missing values and outliers that could skew analysis. Graphing relationships in the dataset also helps spot issues. However, the most common data cleaning tasks involve formatting conversions so numbers, dates, categories, etc., are standardized. This way, time periods, product IDs, regions, etc., match up across data sources.
Cleaning ensures complete, consistent data will flow through predictive models. The fewer real-world noise and errors, the better the models can uncover true patterns and trends. Data preparation for predictive analysis may also require enriching datasets with external information, such as social media sentiments or economic indicators related to what is being predicted.
Statistical Foundations
While modern predictive modeling leverages machine learning algorithms, many still use statistical modeling techniques like linear regression. Regression analysis utilizes historical data to define and quantify relationships between variables called predictors (facts we know) and the predicted outcome (what we want to forecast).
A predictive model can estimate likely outcomes based on current predictor values by studying these statistical relationships in the data. Correlation analysis is also key—this measures how strongly variables move in relation to each other. Understanding these fundamental statistical techniques underlying predictive analytics is key to building models and interpreting predictions.
Importance of Predictive Analytics Across Industries
Predictive analytics uncovers trends and patterns by analyzing volumes of data that we couldn’t see just by looking. These technology-enabled methods of analysis allow businesses to anticipate rather than react. Predictive insights support better short and long-term decision-making.
- Retail: Retailers are leveraging predictive data to forecast purchasing trends. This supports inventory and supply chain optimization. Predictive analytics also streamlines marketing campaigns by modeling customer responses.
- Finance: Financial institutions build predictive models factoring economic trends, past performance, and risk analytics to forecast stocks and mitigate investment risks. Models also help detect fraud.
- Insurance: Insurers analyze risk factors in their predictive models to forecast claim rates and premiums to properly assess policies. This supports accurate underwriting.
- Healthcare: Healthcare providers apply predictive analysis to clinical data to anticipate diagnosis and disease risks, enabling improved preventative care.
Key Techniques in Predictive Analysis
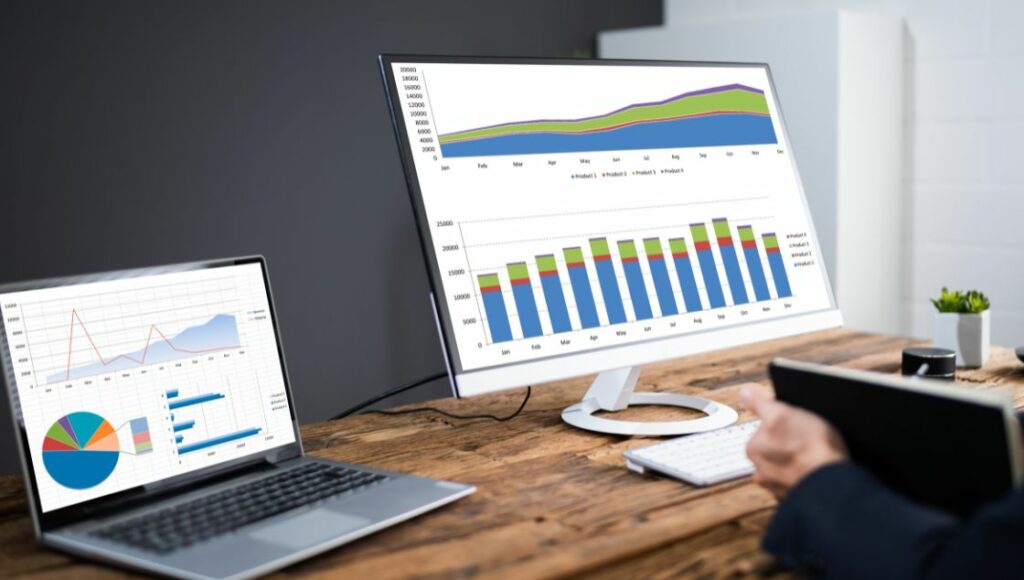
There are various statistical and machine learning techniques used today to develop predictive models. The most common approaches include:
1. Regression Analysis
Regression analysis focuses on defining the relationship between dependent and independent variables. The strength of this relationship is key for predicting likely outcomes. Linear regression is the most popular form, though other forms, like logistic regression, are also widely used.
2. Time Series Analysis
Time series analysis evaluates patterns in historical sequence data over defined time steps. Common techniques include exponential smoothing, ARIMA modeling, and growth curves. The goal is to project patterns forward.
3. Machine Learning Models
Machine learning models uncover complex data relationships and refine predictive capabilities through dynamic algorithms that evolve with ever-growing data inputs without being explicitly programmed. Supervised learning techniques, as well as neural networks, are popular for data forecasting.
4. Deep Learning
Deep learning is an advanced subset of machine learning based on artificial neural networks with multiple layers. This structure enables deep learning models to automatically identify complex connections within large, multifaceted datasets for enhanced predictive modeling.
The Bottom Line
Predictive analytics leverages everything from basic statistical techniques to cutting-edge machine learning innovations. Organizations apply predictive modeling and forecasting across business functions, leveraging the right approach to meet challenges from financial risk to healthcare treatment. In the future, the predictive capabilities unlocked through data analytics will continue to evolve and become more pivotal for data-driven decision-making.