7 Easy Ways to Understand Excel Scatter Plots: Visualize Your Data Effectively!
Scatter plots are one of the best graph types for visualizing relationships between two variables in your Excel data. Plotting one variable on the X-axis and another on the Y-axis allows you to inspect patterns as data points to gain key insights into correlations. This article will explore a scatter plot, its key components, how to prepare your data for plotting, common patterns to recognize, and 7 simple tips to analyze scatter plots like an expert.
What is a Scatter Plot?
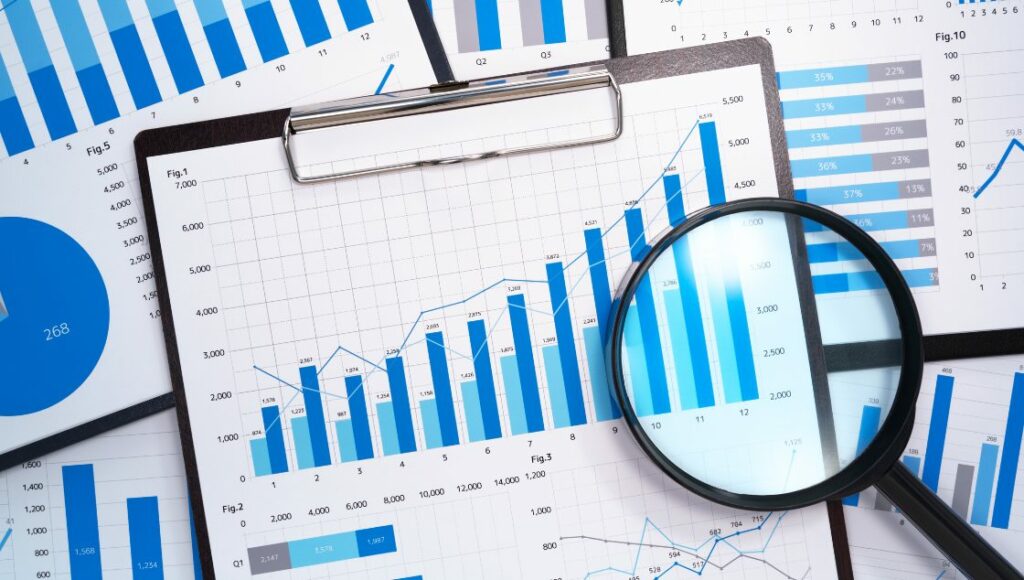
A scatter plot (aka scatter graph, correlation chart) plots pairs of numeric variables to assess if they demonstrate a statistical relationship visually. Each data point on the graph represents an observation or record plotted using an x and y coordinate.
Key Components of a Scatter Plot
The main components of a scatter plot are:
1. X-axis and Y-axis
The x-axis and y-axis are the basic building blocks of a scatter plot. They provide the foundation on which the data points are plotted. The x-axis is mapped to one variable, while the y-axis represents another variable. Typically, the independent variable whose values are not affected by the other variable is placed on the x-axis. For example, in a scatter plot showing the relationship between study time and test scores, study time is the independent variable, while test scores are dependent on it. So, study time would go on the x-axis, and test scores would go on the y-axis.
The scale of the axes reflects the range of data being plotted, and the ticks on the axes provide the measurement units for the numerical data. Properly labeling the axes is important to define the variables memorably. It also helps set the context for interpreting the data points mapped on the axes. Clear axis titles provide instant clarity for the viewer.
2. Data points
The discrete data points mapped on the x and y axes in a scatter plot represent individual records or observations from the dataset. Each data point is placed on the graph based on the x and y coordinate values from the variables plotted on the horizontal and vertical scale. For example, if a student studied for 3 hours (x-axis value) and scored 85 on the test (y-axis), this observation would be plotted as a point with an x value of 3 and y value of 85.
As multiple data points accumulate on the chart canvas, interesting patterns may emerge that give insight into the interplay between the variables.
3. Trend lines
Trend lines help reveal linear or polynomial patterns in the data distribution on a scatter plot. Based on statistical models, Excel fits the “line of best fit” to summarize the general tendency in the data as it progresses from left to right on the chart. The slope and position of the trend line relative to the axes provide clues into the nature of the relationship (positive/negative correlation) and its relative strength.
Trend lines help forecast future outcomes based on historical data. They enhance interpretation and support decision-making by visually bringing out the data’s message.
Setting Up Your Data for a Scatter Plot in Excel
1. Preparing Your Data
It is vital to properly prepare your source data before bringing it into Excel to create a scatter plot. First, assemble the dataset with candidate numeric variables for the x and y axes into an organized table format with column headings. Ensure the raw data is as complete and clean as possible to avoid anomalies in graphing the relationships.
2. Check for Missing Data
Carefully scan your table for any blank cells or gaps which indicate missing records. Depending on analysis needs, decide whether to fill blanks with default zeros or average values. Else consider dropping rows with incomplete data to avoid misshaping relationships. Leaving empty cells may mean they get excluded during mathematical modeling behind correlation analysis.
3. Format Your Data
Verify that the data types and formats in the columns match the variable measurement needs. The x and y axis variables must hold numeric data like numbers or percentages rather than text. Format the columns as Numbers with appropriate decimal places. This enables mathematical operations to determine the best-fit lines and distances. Non-numeric data cannot be plot properly on the quantitative axes.
4. Ensure Numeric Data
Double check that the fields identified to represent the x variable and y variable contain clean numbers that make sense for the real-world parameter they reflect. Text values like categories or labels cannot graph as coordinates on the scatter plot’s axes. The chart will fail to generate or shape patterns accurately if non-numeric data gets assigned to the value columns.
5. Avoid Duplicates
If your table has repeating identical values in the columns designated for plotting, you may notice overlapping data points obscuring each other on the scatter chart. Eliminate redundant duplicate records where feasible before inserting the scatter plot.
7 Ways to Understand Excel Scatter Plots
Identify and Label Your Axes Clearly
Always start by clearly defining the variables or data parameters plotted on each axis. Descriptive axis labels provide critical information for correctly interpreting data patterns and relationship characteristics between the variables. For example, label the x-axis “Study Time (Hrs)” and y-axis “Test Scores (%)”.
Understand the Relationship Between Variables
Examine the overall data point distribution and directional flow to assess whether the x and y variables correlate positively or negatively, if at all. Can you spot any clear linear up/down trends or curves? Strong positive correlations mean y values increase steadily as x values rise. In negative correlations, y falls with increases in x.
Recognize Different Types of Correlations
The scatter plot may reveal more complex nonlinear relationships beyond just positive or negative linear trends. Some tables exhibit grouping or clusters in data, while others have outlying points. Evaluate the appropriate type of trendline e.g. linear, logarithmic, exponential, etc. that best encapsulates the data scatter for what it communicates.
Use Trend Lines to Highlight Patterns
Trendlines mathematically model the general tendencies in the scatter plot, providing a powerful visual summary of directionality and the strength of the correlation between variables. Observe how densely the data points fall around the linear trendline to gauge the spread of values across the central tendency.
Spot and Handle Outliers
Carefully inspect unexpected data points lying far outside the general cluster of points. These may indicate faulty records or experimental anomalies. Statistical analysis of outliers helps inform decisions to include, exclude, or substitute outlier values.
Experiment with Different Data Series
Intriguing insights may surface by plotting different variables against one another or using varied data groupings (e.g., year-wise sales figures). New plots help deeply understand multifaceted real-world relationships.
Practice Interpreting Scatter Plot Results
The more scatter plots you analyze, the stronger your intuitive skills become for spotting patterns and deriving meaning from data visualizations. Think critically to explain correlations witnessed, account for data gaps, and quantify slope direction/steepness.
The Bottom Line
Scatter plots unlock valuable insights by illustrating correlations visually. Mastering key concepts around components, pattern identification, and analysis best practices enables the creation of informative graphs from data tables. Practicing these seven tips will make you an Excel scatter plot pro, capable of discovering data stories and informing decisions confidently through compelling charts. With a reliable data foundation and visual data dexterity, scatter plots transform numbers into actionable business intelligence.