A Step-by-Step Guide to Creating Effective Scatter Plots
A scatter plot, also known as a scatter graph, is a type of graph that uses Cartesian coordinates to display values for typically two variables for a set of data. Scatter plots are useful for detecting any correlations between two quantitative variables and can reveal if they are correlated positively or negatively. This post provides a step-by-step guide to making a scatter plot, from selecting the variables to interpreting the results, showcasing how to plot scatter plots effectively.
Step 1: Decide the two variables
The first and most important step in creating a scatter plot is deciding on the two variables to examine for correlation. There can be many possible variable combinations to choose from, so it is crucial to select variables that are expected to have some meaningful relationship.
For example, if analyzing sales data, variables like revenue vs profit or units sold vs advertising spend would be relevant. Choosing variables without considering their potential relationship can lead to meaningless scatter plots.
It is also important that both variables are numerical/quantitative. Variables like gender or color cannot be directly plotted against each other. Once the two quantitative variables are identified based on the research objective or hypothesis, the data collection process can begin.
Step 2: Collect data
With the variables established, the next step is gathering real data values for these variables from the relevant sample or population. The data collection method depends on the type of variable; an experiment may need to be designed to get measurements if the variables relate to some process. Careful planning is required to collect accurate and meaningful data without errors through surveys, experiments, secondary research, etc.
Data quality is extremely important as garbage in results in garbage out. Sample size, sampling technique, and data measurement methods used need to ensure the collected data represents the target population well. For example, if income vs expenditure is being examined for individuals, more than a small convenient sample will be needed and a larger randomly selected sample is needed.
Step 3: Map the data
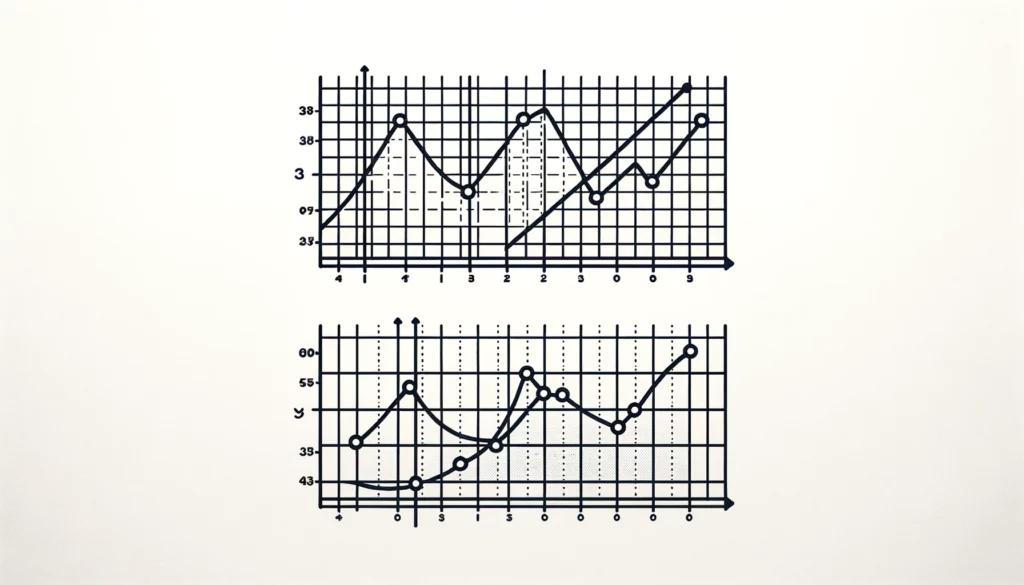
Once data is collected, it must be mapped on a graph with x and y axes. The x-axis will represent one variable, and the y-axis will represent the other variable. Each data point corresponds to an x and y value from the dataset. Modern spreadsheet and plotting software can automatically map data if provided in a clear format, simplifying the process of plotting a scatter graph.
Otherwise, manually listing the x and y values on a grid and marking the points may be needed. After plotting, one can get a visual sense of any apparent relationship or pattern between the variables by observing where most data points lie or if any outliers exist. This preliminary examination of the scatter plot may provide early insights.
Step 4: The line of best fit
To analyze the correlation more objectively, the next step is determining the “line of best fit” for the data points. This line will best summarize/represent the overall relationship between the variables. Finding the line of best fit requires making calculations to draw a line that minimizes the distances between the points and itself.
In the past, this involved complex statistical computations. Now, spreadsheet functions and programs plot the line of best fit automatically. Observation of the points around this line gives an idea of how strongly the variables correlate.
Step 5: Come up with an exact number
After plotting and examining the line of best fit visually, it is important to quantify the correlation between the variables with a numeric measure. This is done by calculating the Pearson correlation coefficient (r), which ranges from +1 to -1.
Values closer to +1 or -1 indicate stronger positive or negative correlation, respectively, while a value close to 0 shows no correlation. Most statistical software output r directly. In Excel, the CORREL formula returns r. This single number provides an objective measure of just how closely related the variables are based on the entire dataset.
Step 6: Interpret the number
The last and most important step is making sense of the correlation coefficient value. As a thumb rule, |r| > 0.5 indicates a reasonably strong/high correlation, 0.3 < |r| < 0.5 a moderate correlation, and |r| < 0.3 a weak correlation. No correlation is shown by r = 0. A perfect positive or negative correlation of +1 or -1 is also assessed.
While a high r value establishes a statistical relationship, it does not prove causation. Other factors need examining, too. For example, two variables may be correlated due to their mutual dependence on some third variable. The correlation direction (positive or negative) also needs explaining. Scatter plots thus provide a starting point to understand how variable relationships work rather than conclude them, highlighting the importance of understanding how to create a scatter plot for accurate interpretation.
Conclusion
Making a scatter plot requires selecting relevant variables, gathering their data values, plotting data points on a graph, finding the line of best fit, calculating correlation coefficient r, and interpreting results meaningfully. Modern tools have simplified the process, but human judgment is still crucial, especially at the start and end. Scatter plots remain a very useful exploratory data analysis technique, highlighting the importance of understanding how to plot scatter plots for effective analysis.