A Comparative Analysis: Mastering Techniques for Effective Data Comparison
Comparing data is crucial for gaining insights. However, not all comparison techniques are created equal. Analysts can perform insightful comparative analyses by mastering best practices for ensuring data quality, applying rigorous quantitative and qualitative methods, and leveraging data visualization. This article explores proven techniques to derive statistically sound, meaningful conclusions from data comparisons.
The Role of Data Quality in Effective Comparison
High-quality data is the foundation of effective comparative analysis. Before diving into advanced analysis techniques, ensuring the data being compared is accurate, consistent, and compatible is critical. Here are some key considerations around data quality when making comparisons:
- Data Accuracy: The data should precisely and correctly measure what it is intended to measure, free of errors or bias. Only accurate data will lead to true insights.
- Data Consistency: The data collection methodology should be consistent across the compared datasets. Differences in how the data was gathered can obscure real differences.
- Compatibility: The data must contain comparable metrics measured in the same ways. Comparing apples to oranges will not yield meaningful insights.
- Sufficient Sample Size: The data must have a large enough sample size to be representative of the population. Small samples may be misleading.
- Recent Data: The data periods should match when comparing over time. Comparing old data to new data can reflect changes in methodology.
- Common Units: The data should be standardized to common formats, units of measure, currencies, etc., to allow for an apples-to-apples comparison.
By carefully evaluating data quality on these factors prior to analysis, you can have greater confidence in the insights derived and avoid misleading or meaningless conclusions. Cleaning, normalizing, and aligning data sets before comparing is time well spent.
Techniques for Effective Data Comparison
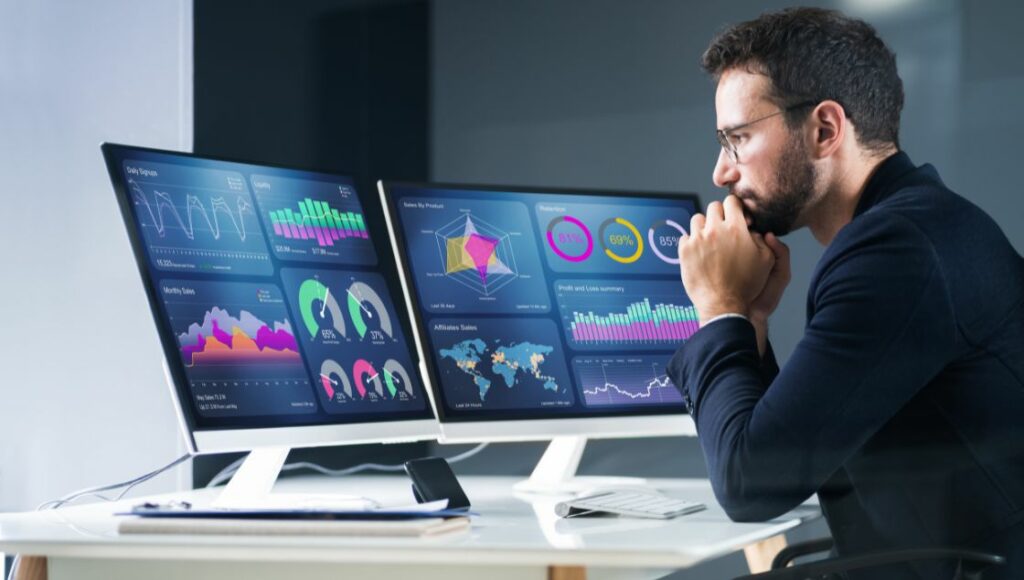
Once you have confirmed the quality of the data, you can use a range of quantitative, qualitative, and visual techniques to perform insightful comparative analyses.
1. Quantitative Comparison Techniques
Statistical analysis is essential for numeric data comparison. Common methods include:
- T-tests – Compare the means of two groups to see if they are statistically different. T-tests determine the probability the difference is due to chance.
- ANOVA – Compare means across multiple groups. ANOVAs assess differences in group means relative to variability within the groups.
- Correlation analysis – Measure the strength of association between two variables to see if they move in tandem. Correlations range from -1 to 1.
- Regression analysis – Evaluate the predictive relationship between independent and dependent variables. Regression quantifies cause and effect.
These quantitative techniques allow you to make statistically valid comparisons on numeric data that account for natural variation and chance. The results provide mathematically sound insights into differences, associations, and causal relationships.
2. Qualitative Comparison Techniques
For textual, survey, interview, and observational data, qualitative comparative methods enable thematic analysis:
- Content analysis – Systematically evaluate the content frequency and associations to derive insights.
- Thematic analysis – Discover underlying themes and concepts within qualitative data through coding.
- Narrative analysis – Assess patterns in participant stories and experiences to learn motivations.
Qualitative techniques uncover meaningful themes and explanations for how groups experience phenomena differently based on their own words and perspectives.
3. Visual Comparison Techniques
Visualizing data amplifies insights from statistical and thematic comparisons through impactful graphical representation. Useful visual techniques include:
- Charts: Bar, line, and scatter plots effectively convey statistical differences, correlations, and regression results.
- Heat maps: Color-coded tables emphasize patterns in complex data sets at a glance.
- Dashboards: Combining multiple charts, metrics, and filters facilitates interactive data comparison.
Visual data comparison promotes an intuitive understanding of complex comparative findings. Graphics make recognizing differences, associations, and patterns easy for broad audiences.
By combining quantitative, qualitative, and visual comparison techniques, you can derive richer, multidimensional insights from your data. Choose techniques aligned to the type and purpose of the comparison. Use data visualization to ensure the findings are consumable.
Advanced Tools and Software for Data Comparison
Sophisticated tools and software are available to streamline and enhance comparative data analysis. Key solutions include:
- Spreadsheets: Microsoft Excel and Google Sheets provide built-in charting plus statistical add-ons like the Analysis ToolPak.
- Statistical software: SPSS, SAS, and R, offers expanded analytical capabilities, such as ANOVA, regression, and factor analysis.
- Data visualization: Tableau, Power BI, and Qlik allow interactive visualization and dashboarding.
- AI and machine learning: Solutions like Watson Compare and Decisions enable automated data comparison using algorithms.
These advanced tools remove manual work in cleaning, aligning, analyzing, and visualizing data comparisons. They provide automation, analytics, and ease of use that facilitate frequent, impactful data comparisons using best practice techniques. With the right tools, anyone can derive actionable insights through data comparison.
The Bottom Line
Comparing data sets is essential for understanding differences, testing theories, and identifying opportunities. Data analysts can deliver significant business value through insightful, statistically sound analysis by honing techniques for effective comparison accompanied by the right enabling tools. With valid data inputs and rigorous quantitative, qualitative, and visual comparison methods, meaningful conclusions can be drawn to drive data-driven decision-making.